How Innovior is helping businesses navigate the hype with its Applied AI approach
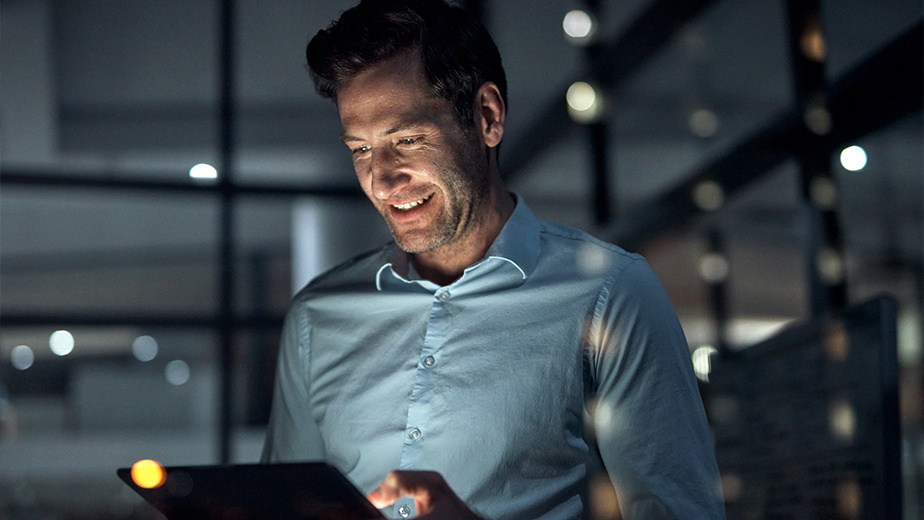
Despite this massive investment and hype, most AI projects have failed to materialise into compelling business value, with some sources estimating that over 80% of AI projects fail3. In this article, we will explore what has caused this disconnect between AI hype and AI results and how Innovior is helping clients achieve genuine AI value through our Applied AI approach.
Navigating the pitfalls of AI
Why do so many AI projects fail and how can future AI adopters avoid a similar fate? Here are four key pitfalls that business leaders must navigate when pursuing an AI solution.
- Choosing the wrong AI tool: we use the catch-all term Artificial Intelligence (AI) to describe any digital solution that can emulate complex human behaviour e.g. predicting, deciding, creating and understanding. However, AI is comprised of a multitude of distinct Machine Learning (ML) algorithms which vary greatly in terms of design, capability, and suitability for particular problem types. When considering a business solution, business leaders must not only ask themselves “Is AI the right tool for the job?”, but they should also ask “What particular AI solution best fits my business problem?”
- Lack of data readiness: data is the lifeblood of AI. Without high-quality, relevant data, AI models cannot produce meaningful insights. Before diving into the AI arena, business leaders need to clearly understand what business data is available, where it will come from, who will manage it over time, what biases might exist in the dataset and what steps must be taken to make the data “AI-ready”.
- Ignoring the limitations of AI: AI is not a panacea that can solve every business problem. Sometimes AI can lead to biased predictions, false information, poor customer experiences and other negative business outcomes. The potential limitations of an AI solution must be considered when deciding on a solution approach.
- Siloed AI experimentation: AI in itself doesn’t deliver value as a stand-alone technology, it needs to be integrated as part of a fully thought-through solution that considers how the business process will work in the future. Systems thinking is needed to make sure that you don’t end up with AI that can’t be used to drive tangible outcomes.
Applied AI – Innovior’s approach to navigating the AI hype
At Innovior, our lean process DNA allows us to help organisations define the right problems to solve with AI, prepare for living with AI and deploy effective AI solutions into production. We’ve been delivering complex technology solutions to customers for the best part of a decade and that experience has never been more relevant than now when helping customers avoid the common pitfalls of AI.
Here’s how we do it:
Step 1: Identify a compelling business problem
We start by understanding the specific challenges and opportunities within an organisation. This ensures that AI is applied where it can genuinely add value. We then help build the business case to support the necessary investment to move the best ideas forward.
Step 2: Assess the suitability of AI
Not every problem requires an AI solution. We evaluate whether AI is the right tool for the job, considering factors such as complexity, data availability, training requirements and the potential for automation.
Step 3: Select the right models and software
The choice of AI models is critical. We select models that are best suited to the problem at hand, balancing accuracy, interpretability and scalability. We help make the tradeoff decision between build vs buy which is a key consideration for getting AI ready in a cost-effective manner.
Step 4: Get ‘AI-ready’
Data is the lifeblood of AI. We have a rigorous method for getting data ready which encompass data collection, cleaning, integration and governance, ensuring that AI models are fed with high-quality data.
Step 5: Build, deploy and support the solution
We set up the infrastructure, train the models and deploy the full-scale solutions into production environments. We help train AI champions, support the onboarding of users and provide post-deployment technical performance assessments and insights.
Applied AI in action
Through our Applied AI approach, we have delivered substantial results for our clients. Here are a few recent examples:
- Predictive maintenance in manufacturing: by implementing AI-driven predictive maintenance, we helped a manufacturing client reduce downtime by 30%. Our models analysed sensor data to predict equipment failures before they occurred, allowing for timely maintenance and minimising production disruptions.
- Customer service agent assistant: for a leading energy distribution company, we developed an AI-powered chatbot that handled 70% of customer enquiries, improving response times and customer satisfaction. This automation enabled the company's human agents to focus on more complex issues, enhancing overall service quality.
- Intelligent policy and procedure knowledgebase: for a large private hospital operator and healthcare provider group, we developed a generative AI-powered chatbot that allowed nurses to ask questions about internal policies and procedure documents in natural language. This saved each user 30 minutes per day in wasted search time which was re-directed towards spending more time caring for patients.
Conclusion
The hype around AI is undeniable, and its potential to revolutionise various industries is immense. The best way businesses can leverage this trend is the first identify and qualify the business problems that AI can help solve, making sure they build a robust business case to support the investment needed. The next step is to start with a series of low-risk pilots which make collaboration easier and lower the barriers to success. The momentum built from the early pilots earns crucial trust from business leaders to keep scaling the use of AI across the enterprise.
Reference:
[1] https://ecommercenews.com.au/story/australian-cios-increase-ai-investment-amid-application-sprawl-concerns
[2] https://www.statista.com/forecasts/1474143/global-ai-market-size#:~:text=The%20market%20for%20artificial%20intelligence,billion%20U.S.%20dollars%20in%202030
[3] https://hbr.org/2023/11/keep-your-ai-projects-on-track