The generative AI dilemma - is it suitable for all businesses?
Technology hype does not come much bigger. From the moment OpenAI shared its latest conversational chatbot with the world on November 30, 2022, the world got very excited about ChatGPT. Within two months, it had gained more than 100 million users to become the fastest-growing consumer software application in history1. While that record has since been surpassed by Twitter rival Threads2, few of us will ever forget how much people were chatting about ChatGPT in early 2023.
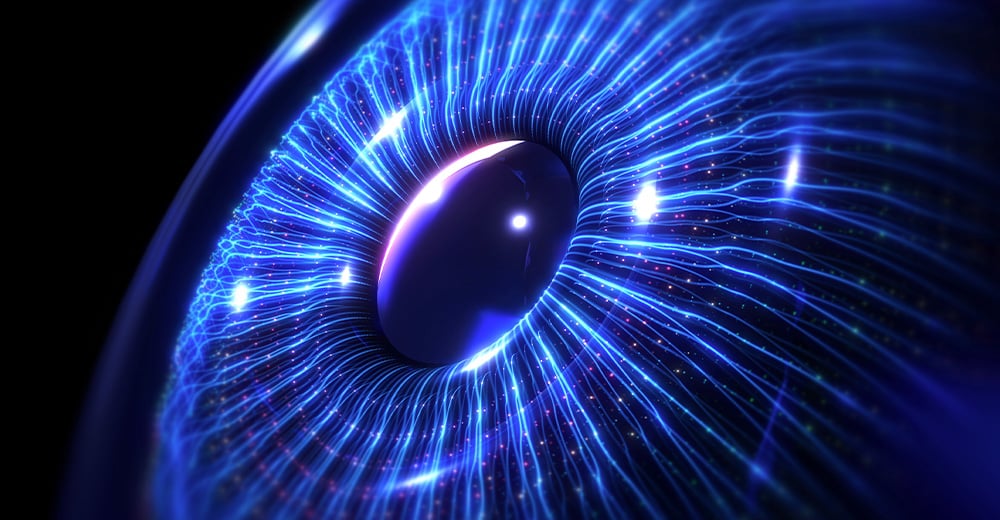
Among those people were business leaders desperate to embrace the technology powering the ChatGPT revolution. Generative AI may have its origins in the 1960s3 but the incredible success of OpenAI’s application saw organisations rushing to invest in their own projects to speed up or improve their existing processes and keep one step ahead of competitors. For proof of the gold rush, look no further than an Axios report that showed funding for generative AI projects soared from $613 million in 2022 to $2.3 billion in 20234.
Given such hype, one cannot blame businesses for pursuing their own generative AI projects but it has never been more important for decision-makers to remember there is often a stark contrast between hype and reality. While the technology undoubtedly boasts huge potential, it is equally the case that there are very real limitations that may result in organisations wasting considerable time and money that would have been better invested elsewhere.
What is generative AI?
Generative AI refers to deep-learning models that use the data they are trained on to generate high-quality text, images and other content. Based on ‘models’ of statistical probability and machine learning algorithms, the technology is used to create content (as opposed to other forms of AI that are used for different purposes) and, by doing so, can speed up, scale or improve business processes.
What are the risks and limitations of generative AI?
Generative AI is a powerful tool but, like any technology, it has drawbacks that should be considered before investing in the development and deployment of such solutions.
- Biased outputs: with generative models trained on data sets, one of the key concerns for users is that any biases contained within those data sets will be reflected in the output of the model. This has been evidenced in chatbots that demonstrate political bias and image generators that have produced outputs that amplify racial and gender stereotypes. While some might like to believe that tapping into more training data would mean less bias, the opposite has often been proven to be the case as larger volumes merely reinforce existing biases.
- Inaccurate information: amid the celebratory headlines about ChatGPT, more measured voices were quick to raise serious concerns about ‘hallucinations’ – that is, the potential for generative AI models to confidently rely on false training data. There is no doubt that text-based generative AI models can be easily led, as evidenced by the New York lawyer who used ChatGPT to prepare for a court case but was caught out when his brief cited several fake cases that included “bogus judicial decisions with bogus quotes and bogus internal citations”5. This threat of incorrect information is why businesses should avoid using generative AI for customer-facing interactions without human oversight.
- Lack of new ideas: for all its jaw-dropping capabilities, there is one reality that generative AI cannot escape. With most models based on pre-existing data and rules, it is simply unable to generate new ideas or solutions. This lack of creativity is because generative AI relies on its training data and therefore can only produce results that are similar to what has been previously done. ‘Thinking outside the box’ is not on the agenda and while there are obvious advantages to the technology, there is a long way to go before generative AI can match the intelligent thinking of humans.
- Privacy concerns: data security should be one of the highest priorities for any modern business, which is why they should be concerned about the risks of using public generative AI models. With no guarantee over how data that is inputted will be used, let alone how access to it will be controlled, the chances of being exposed to privacy breaches are immense. Providing information to a public generative AI chatbot is a risky business as the last thing one wants is for their confidential or personal details to be compromised – let alone that of their customers.
- Time and money: while the likes of ChatGPT have been marketed for their simplicity, the reality is there is an enormous engine behind the solution. Between specialised technology and highly skilled staff, generative AI can be incredibly expensive to implement – and that is without factoring in ongoing maintenance. Many organisations have already been caught out by trying to realise their generative AI ambitions with limited budgets, while small and medium-sized businesses have found the length of time needed to train and deploy solutions is a major impediment for success.
How to approach generative AI adoption?
This is not an article about avoiding generative AI. Rather, it is a warning to take care when deploying it. Before blindly throwing money at pilot projects, businesses need to research and understand its limitations and take appropriate steps to ensure any solutions or tools that are developed deliver what they are designed to do.
A key step is to tap into the knowledge and experience of people with a proven track record of generative AI innovation. This was the case for a leading Australian telecommunications and telecom agency that faced a daily battle to manually review, summarise and categorise up to 700 industry and competitor-relevant online articles. Knowing the power of generative AI, it envisaged a solution that would automatically scan those feeds, collate and send a finalised report to users.
However, rather than go it alone, the agency turned to Innovior, which has used a combination of intelligent automation and generative AI to deliver a fully automated report that can be sent out to more than 4,000 users. The partnership has delivered several outstanding results including a service that is two to three times more cost-effective than legacy insight software products, a boost in employee satisfaction and report insights that have been used to successfully identify market and competitor threats earlier than previously possible.
Summary
Like any major investment, the pursuit of generative AI requires a concerted effort to mitigate risks and maximise opportunities. Effective prompt engineering, consideration of ethics, ongoing reviews of training data and a healthy dose of professional scepticism are all advisable, not to forget working with a tech company that has previously trodden the generative AI path. Ultimately, it is all about doing everything one can to ensure their business is talked about as a generative AI success story rather than becoming a case study of what not to do.
Looking for more inspiration of what is possible when experts put their minds to generative AI solutions? Discover how the likes of healthcare providers, universities and insurance groups are using the power of technology to achieve business growth.
Reference:
[1] ChatGPT continues to be one of the fastest-growing services ever - The Verge
[2] Meta's Twitter rival Threads surges to 100 million users faster than ChatGPT | Reuters
[3] History of generative AI (toloka.ai)
[4] Metaverse funding plummets as investors favor generative AI (axios.com)
[5] Lawyer cites fake cases generated by ChatGPT in legal brief | Legal Dive